In the realm of machine learning (ML) and artificial intelligence (AI), deploying models to production is the final, crucial step in the lifecycle of model development. This process does not only involve the deployment itself but also rigorous testing in controlled environments and a systematic transition to production settings. This article explores the significance of deploying models to production, the methodology for testing them in a staging environment, and leveraging Domino’s capabilities, including Model Sentry, to ensure these models operate effectively in production with a human-in-the-loop review and approval process.
Model deployment is the phase where theoretical data science transitions into practical application, allowing businesses to start reaping the tangible benefits of their investments in AI and ML. Models, no matter how well they perform in a controlled test environment, only deliver value when they are integrated into real operational systems where they can influence decision-making and automate processes.
Deploying models to production environments allows them to interact with real, live data. It also allows for the gathering of feedback necessary for iterative improvement, ensuring the model stays relevant as market dynamics and data patterns evolve.
Consider the following best practices when you deploy your models:
Test in a controlled environment
Before a model is fully deployed into production, it is essential to deploy it in a test or staging environment. This environment closely mirrors the production environment but does not affect the actual operational processes or workflows.
-
Validation: In this stage, the model is tested against a set of predefined criteria to validate its accuracy, efficiency, and reliability.
-
Integration testing: The model’s integration with other systems and workflows is tested to ensure that there are no integration issues.
-
Load testing: This tests the model’s performance under various loads to ensure that it can handle expected transaction volumes.
Using Domino’s advanced ML lifecycle management capabilities, transitioning models from a staging to a production environment involves structured steps and checks powered by Domino Model Sentry. Model Sentry allows for the registration of models in the Model Registry, followed by a human-in-the-loop review and approval process, powered by Domino, before deployment to production. Finally, Domino Model Monitoring continuously tracks model performance to ensure they are effectively meeting your business goals.
Separate environments
It’s important to have a controlled process to separate changes in a Dev
or Staging
environment before going to Production
. This can help prevent unintended consequences. However, there are multiple ways to achieve this.
-
Separate data planes: Domino supports a hybrid, multi-cloud architecture called Domino Nexus. Domino Nexus enables preparing data or training models in one data plane or region and deploying to another, or using many different data planes for training and deploying models. As a best practice, we recommend that you use separate data planes for
Dev
,Staging
, andProduction
to ensure the isolation of resources and tight control over who can make changes in production for security reasons. To learn more, see Domino Nexus. -
Node pool isolation: Domino runs on Kubernetes-based infrastructure, both in the cloud and on-premise. If you’d like to have separate
Dev
andStaging
environments, which are collocated in the same Kubernetes cluster, it is possible to create separate Kubernetes Node Pools to ensure complete isolation of the virtual machines and containers that get allocated. Therefore, you can create a node pool forDev
and a separate node pool forProduction
. To learn more, see node pools in Domino.
Domino supports different aspects of model governance:
-
Model cards: Domino uses model cards to track model lineage to create a system of records that helps you evaluate a model’s accuracy, fairness, compliance, and auditability. Project owners can collaborate with multiple stakeholders on these cards, ensuring security with fine-grained access control. Use Domino’s integrated model review process to ensure that production models meet organizational policies and regulations, and avoid unintended business impacts.
-
Review process: Before a model is transitioned to production, it undergoes a review process where domain experts, data scientists, and stakeholders analyze its performance metrics and outcomes in the staging environment.
-
Approval process: After thorough review, the model must be approved by authorized personnel. This step ensures accountability and adherence to organizational standards and regulatory requirements.
-
Gradual rollout: Instead of a full-scale deployment, models are often rolled out gradually. This allows teams to monitor the impact of the deployment and make the necessary adjustments before full deployment.
-
Feedback loop: Establish a feedback mechanism to continuously collect insights on the model’s performance in the production environment. This data is crucial for ongoing improvement and future iterations of the model.
Accountability: Domino ensures clear role and responsibility assignment with its access control policy based on attributes and actions associated with model creation and management.
Transparency and reproducibility: Domino records the exact code, Environment, Workspace settings, datasets, and data sources for each experiment and associates them with the published model. This data is easily accessible in every model card to help you understand exactly what went into a model and how to reproduce it.
Accuracy and monitoring: Domino offers prebuilt and customizable environments to let users embed best-in-class explainability solutions to report model fairness and bias for every training job. Customizable model cards can present these reports, giving all collaborators visibility into critical metrics like feature importance, cohort impact, and bias evaluations. Additionally, registered models deployed to a Domino endpoint can take advantage of automatic model drift detection to ensure continuous accuracy.
-
Initiate the review: Project owners and collaborators can request a review from reviewers (fellow project members). The requester creates a note for the reviewers and sets the desired stage for the model, for example,
Staging
orProduction
. -
Review notification: Once initiated, reviewers receive a Domino notification informing them of the model review request. Reviewers can follow the link provided in the notification message that takes them to the model card details for the specific model version.
-
Review the model: Reviewers examine the sections of the model card relevant to their role and responsibility. Reviewers can test the model via the embedded Domino endpoints, explore the experiment details, or even open a Workspace to dive deeper into the training context. The review workflow continues until all reviewers are satisfied and approve the model. Once all reviews are approved, the model automatically transitions to the target stage.
Domino supports custom stages for model versions. You can expand on the default Staging
and Production
states of MLflow, giving you the flexibility to mold the review process to your needs. You must be a Domino admin or a project owner to define custom stages.
For example, you could add model version stages like Pre-Production
or Staging-2
to add more granularity to your model reviews.
Note
|
|
Global stages settings
Domino admins can modify global custom stages for all Projects in the organization.
To set global stages:
-
Go to the Admin Panel > Platform settings > Configuration records.
-
Add a com.cerebro.domino.registeredmodels.stages record with the desired set of comma-separated global custom stage values.
-
Restart the services to apply the changes.
Project stage settings
A Project owner can override the global custom stages for a specific Project. The project-level custom stages apply to all model versions in the Project.
For example, when you start with the MLflow defaults (Staging
and Production
), an admin can override these system defaults with global custom stages (Staging
, Pre-Production
, and Production
). A Project owner can override the global custom stages with project-level custom stages (Staging-2
, Pre-Production-2
, and Production-2
).
To set Project stages:
-
Go to Project > Project Settings > Models.
-
Edit the Custom model version stages.
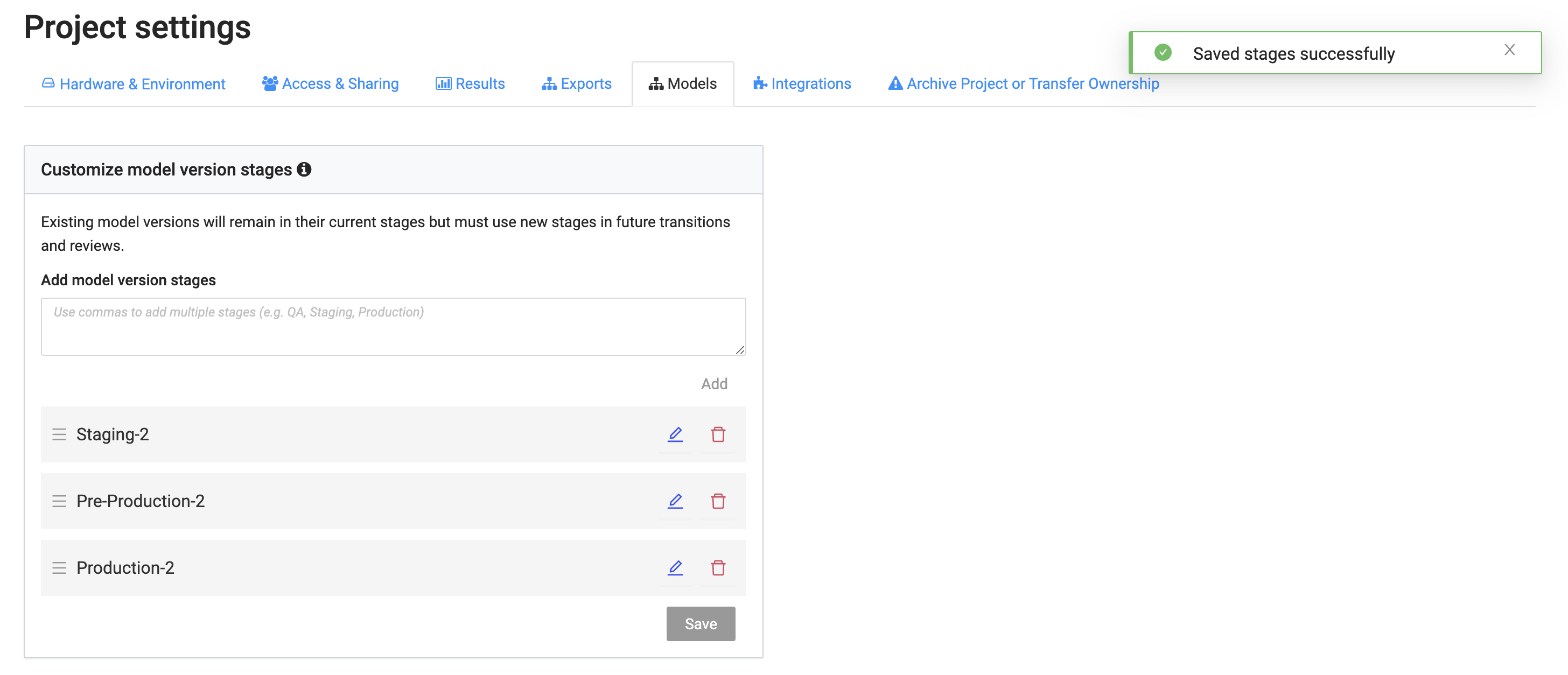
See a log of previous stage transitions, review requests, and review responses in the activity log. Find links to a model version’s activity log in the Reviewers section of its model card or in the Activity column of the version history table.
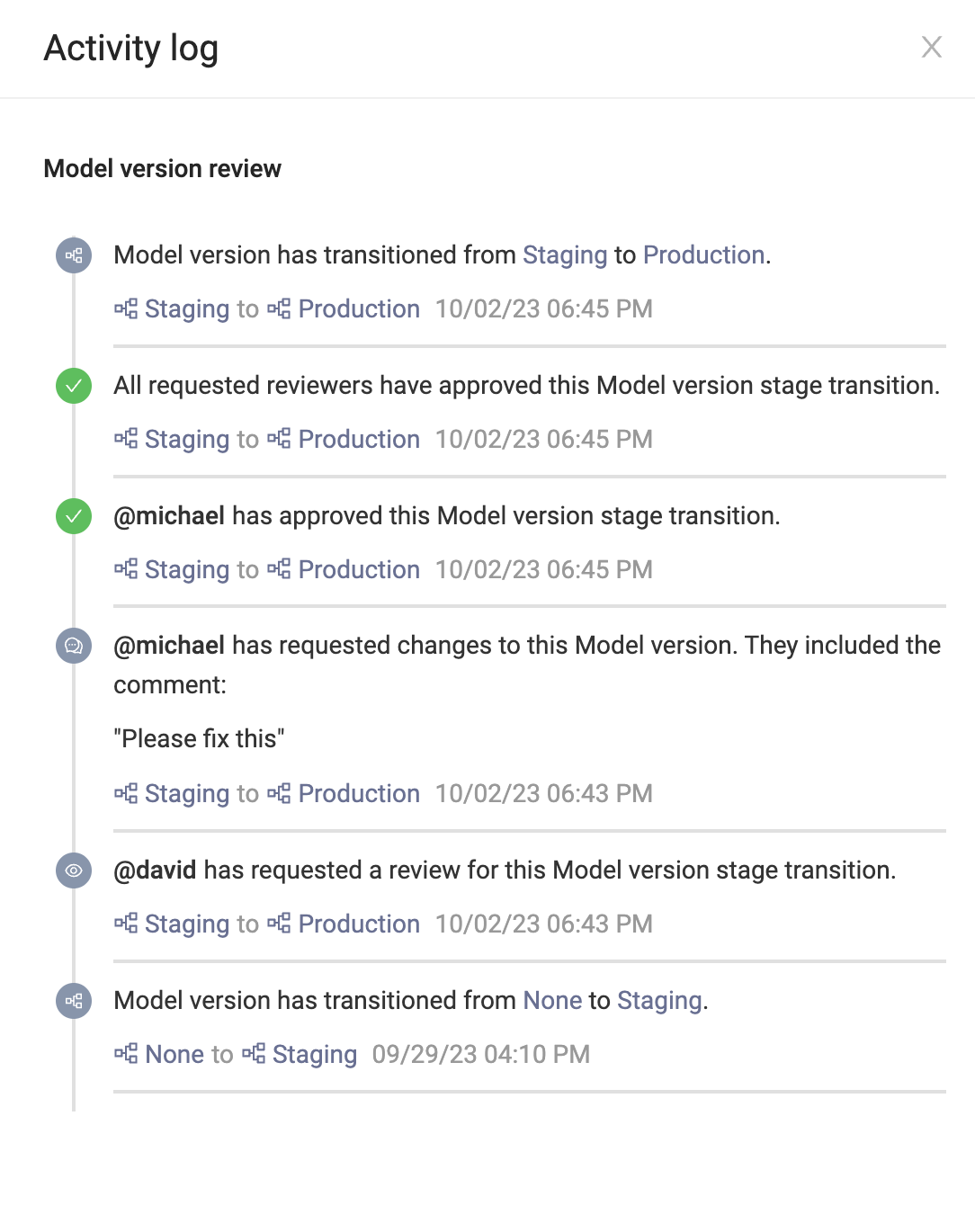
-
Export a model as a container to serve as a prediction endpoint in your own production environment.
-
Export via one of our integrated solutions for SageMaker, NVIDIA Fleet Command, or Snowflake.